NLP - Basic Text Processing
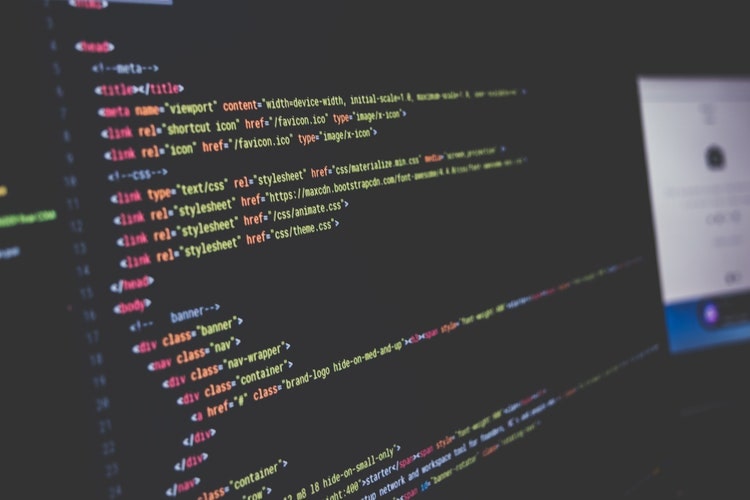
Machine learning algorithms cannot work with raw text directly and therefore the text must be converted into numbers. (specifically, vectors of numbers.)
Pre-Processing in NLP: Pre-processing is one of the most important steps to prepare text documents before any modeling.
following are the most widely used method:
Text Normalization Refers to a series of task for uniforming the test for processing.
- Case Conversion
Convert all text to lower or upper case.
1 2 3 4 5 6 7 8 9 10 11 12 13 14 15 16 17 18 19 20 21 22 23 24
paragraph="A paragraph is a brief piece of writing that's around seven to ten sentences long. It has a topic sentence and supporting sentences that all relate closely to the topic sentence. The paragraph form refers to its overall structure, which is a group of sentences focusing on a single topic." def paragraph_to_lower_or_upper(paragraph,case): if (isinstance(paragraph, str) and case in ["upper","lower"]): if case=="lower": paragraph=paragraph.lower() return paragraph elif case=="upper": paragraph=paragraph.upper() return paragraph else: print("Wrong case or Data format") paragraph_to_lower_or_upper(paragraph,"lower") paragraph_to_lower_or_upper(paragraph,"upper") Output: #Lower Case: a paragraph is a brief piece of writing that's around seven to ten sentences long. it has a topic sentence and supporting sentences that all relate closely to the topic sentence. the paragraph form refers to its overall structure, which is a group of sentences focusing on a single topic. #Upper Case: A PARAGRAPH IS A BRIEF PIECE OF WRITING THAT'S AROUND SEVEN TO TEN SENTENCES LONG. IT HAS A TOPIC SENTENCE AND SUPPORTING SENTENCES THAT ALL RELATE CLOSELY TO THE TOPIC SENTENCE. THE PARAGRAPH FORM REFERS TO ITS OVERALL STRUCTURE, WHICH IS A GROUP OF SENTENCES FOCUSING ON A SINGLE TOPIC.
-
Punctuation removal
Removing punctuations such as : ? , ! ,’ ; - from sentences.
1 2 3 4 5 6 7 8 9 10 11 12 13
sentence=". Period ? Question Mark ! Exclamation Mark , Comma ' Apostrophe : Colon ; Semicolon - Dash - Hyphen" def remove_punctuation(sentence): import re sentence_clean = re.sub(r'[^\w\s]', '', sentence) return sentence_clean remove_punctuation(sentence) Output: ' Period Question Mark Exclamation Mark Comma Apostrophe Colon Semicolon Dash Hyphen'
- Stopwords removal
Stop words are some common words which don’t contain much information such as the, a, this
Better to remove these words from the text.
1 2 3 4 5 6 7 8 9 10 11 12 13 14 15 16
words_list=['It', 'has', 'a', 'topic', 'sentence', 'and', 'supporting', 'sentences', 'that', 'all', 'relate', 'closely', 'to', 'the', 'topic', 'sentence'] def remove_stopwords(words_list): from nltk.corpus import stopwords words_clean = [] for word in words_list: if word not in stopwords.words('english'): words_clean.append(word) return words_clean remove_stopwords(words_list) Output: ['It','topic','sentence','supporting','sentences','relate','closely','topic','sentence']
Tokenization Spliting of text or sequence of character into smaller chunks called as tokens. Types of tokenizer :
-
Sentence tokenizer : splitting a paragraph into individual sentences. Split at ‘. {Capital letter}’
1 2 3 4 5 6 7 8 9 10 11 12 13 14 15
paragraph="A paragraph is a brief piece of writing that's around seven to ten sentences long. It has a topic sentence and supporting sentences that all relate closely to the topic sentence. The paragraph form refers to its overall structure, which is a group of sentences focusing on a single topic." def sentence_tokens(paragraph): from nltk.tokenize import sent_tokenize sentence_tokens_list=sent_tokenize(paragraph) return sentence_tokens_list sentence_tokens(paragraph) Output: ["A paragraph is a brief piece of writing that's around seven to ten sentences long.", 'It has a topic sentence and supporting sentences that all relate closely to the topic sentence.', 'The paragraph form refers to its overall structure, which is a group of sentences focusing on a single topic.']
- Word tokenizer : splitting a sentence into individual words.
Split at ‘Space’
1 2 3 4 5 6 7 8 9 10 11 12
sentence="A paragraph is a brief piece of writing that's around seven to ten sentences long." def word_tokens(sentence): from nltk.tokenize import word_tokenize word_tokens_list=word_tokenize(sentence) return word_tokens_list word_tokenize(sentence) Output: ['A','paragraph', 'is','a','brief','piece','of','writing','that',"'s",'around', 'seven', 'to','ten','sentences','long','.']
Stemming Refers to a crude heuristic process that chops off the ends of words to get base or root word. Output stem can often be non-existent word.
1
2
3
4
5
6
7
8
9
10
11
12
13
14
15
16
words_list=['It', 'has', 'a', 'topic', 'sentence', 'and', 'supporting', 'sentences', 'that', 'all', 'relate', 'closely', 'to', 'the', 'topic', 'sentence']
def stem_words(words_list):
from nltk.stem import LancasterStemmer
stemmer = LancasterStemmer()
words_stem = []
for word in words_list:
stem = stemmer.stem(word)
words_stem.append(stem)
return words_stem
stem_words(words_list)
Output:
['it', 'has', 'a', 'top', 'sent', 'and', 'support', 'sent', 'that', 'al', 'rel', 'clos', 'to', 'the', 'top', 'sent']
Lemmatization Remove inflectional endings and return the base, which is known as the lemma. Output lemma can be looked up in a dictionary.
1
2
3
4
5
6
7
8
9
10
11
12
13
14
15
16
words_list=['It', 'has', 'a', 'topic', 'sentence', 'and', 'supporting', 'sentences', 'that', 'all', 'relate', 'closely', 'to', 'the', 'topic', 'sentence']
def lemmatize_words(words_list):
from nltk.stem import WordNetLemmatizer
lemmatizer = WordNetLemmatizer()
words_lemma = []
for word in words_list:
lemma = lemmatizer.lemmatize(word, pos='v')
words_lemma.append(lemma)
return words_lemma
lemmatize_words(words_list)
Output:
['It', 'have', 'a', 'topic', 'sentence', 'and', 'support', 'sentence', 'that', 'all', 'relate', 'closely', 'to', 'the', 'topic', 'sentence']